How to Become a Data Scientist
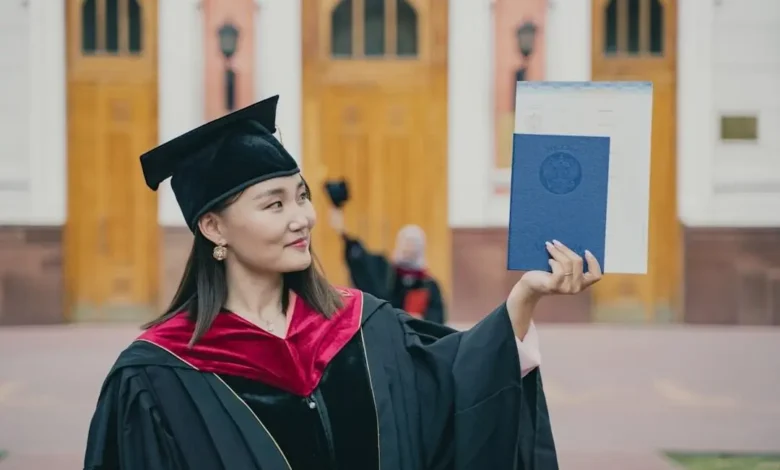
Becoming a data scientist entails continuously pursuing knowledge and expertise across various technical domains. As industries increasingly rely on data-driven decisions, the demand for skilled data scientists has surged, prompting many professionals to seek specialized education to enter or advance in this dynamic field. Keep reading as we uncover the steps and strategies that can propel you toward a fulfilling career in data science.
Exploring Data Science Mastery through Graduate Certificate Programs
Aspiring data scientists face a crucial decision in their journey toward expertise: selecting the optimal educational pathway to acquire essential knowledge and skills. Among the myriad options available, pursuing a certificate in data science stands out as a focused and efficient entry point into the field.
Professionals aiming to enhance their expertise may find graduate certificate programs especially advantageous due to their specialized curriculum. Designed to provide practical understanding in areas such as data analytics, machine learning, and statistical methods, these programs lay a solid foundation for graduates to analyze complex datasets and derive actionable insights.
In today’s competitive job market, credentials play a pivotal role in distinguishing candidates. A Graduate Certificate in Data Science enhances one’s resume, opening doors to valuable networking opportunities with industry experts and peers. This advantage benefits individuals transitioning into data science from diverse professional backgrounds.
Building a Strong Foundation in Mathematics and Statistics
The journey to data science mastery commences with a robust grounding in mathematics and statistics. Students must cultivate an understanding of algorithms, probability, and statistical models as they form the bedrock upon which data analysis is built. Mastery in these areas allows for translating raw data into meaningful, actionable knowledge.
Engagement with advanced mathematics sharpens problem-solving skills, which is essential for tackling the complexities inherent in big data. Courses focusing on linear algebra, calculus, and discrete mathematics prepare students for the analytical rigors of data science. This mathematical toolkit is critical for developing algorithms and models tailored to specific data-driven questions.
Statistics play a pivotal role in interpreting the patterns and trends that data reveals. With a strong command of statistical principles, aspiring data scientists are equipped to infer conclusions and make predictions authoritatively. This statistical competency empowers professionals in the field to provide clarity and direction in a sea of information.
Developing Programming Skills through Secondary Education
The path to becoming a data scientist invariably involves honing programming aptitude, a pivotal skill often cultivated during secondary education. In classrooms, students encounter their first programming languages, setting the stage for future proficiency in Python, R, or SQL, quintessential in data science. This foundational exposure is essential, providing the basis for sophisticated data manipulation and analysis techniques used later in their careers.
Secondary education institutions play a key role in nurturing the programming capabilities of budding data scientists. Through structured coursework, educators instill practices promoting clean, efficient code writing, which is a crucial skill in a data scientist’s repertoire. Educators also emphasize debugging and logical thinking, which are indispensable when developing complex data models.
Emerging data scientists benefit from the early development of programming skills as they enable the exploration of real-world datasets and the construction of predictive models. Such practical applications of programming during formative years cement a skill set that is in high demand across various industries. Investing in this skill set is a strategic step towards a versatile and successful career in data science.
Leveraging Internships and Research Opportunities for Early Experience in Data Science
Securing an internship is a significant step for individuals aiming to become data scientists, as it offers a glimpse into the industry’s workings. By working closely with experienced professionals, interns can apply theoretical knowledge to practical challenges, bridging the gap between academic learning and real-world applications. These internships often provide the first platform for demonstrating and refining technical skills.
Research opportunities present another avenue for acquiring hands-on experience in data science. Participating in research projects allows students to delve deeply into specific areas of interest, whether machine learning, data mining, or predictive analysis. Engaging in research cultivates critical thinking and provides evidence of one’s capability to approach data-driven questions creatively.
Early exposure to data science through internships and research positions enhances one’s practical skill set and offers valuable industry connections. These professional relationships can lead to mentorships, collaborations, and even employment opportunities post-graduation. Proactively seeking these experiences sets the stage for a successful transition into the data science field.
Overall, the journey to becoming a data scientist is multifaceted, requiring a combination of specialized education, foundational knowledge, and practical experience. By navigating this path strategically, individuals can position themselves for success in the dynamic and ever-evolving field of data science.